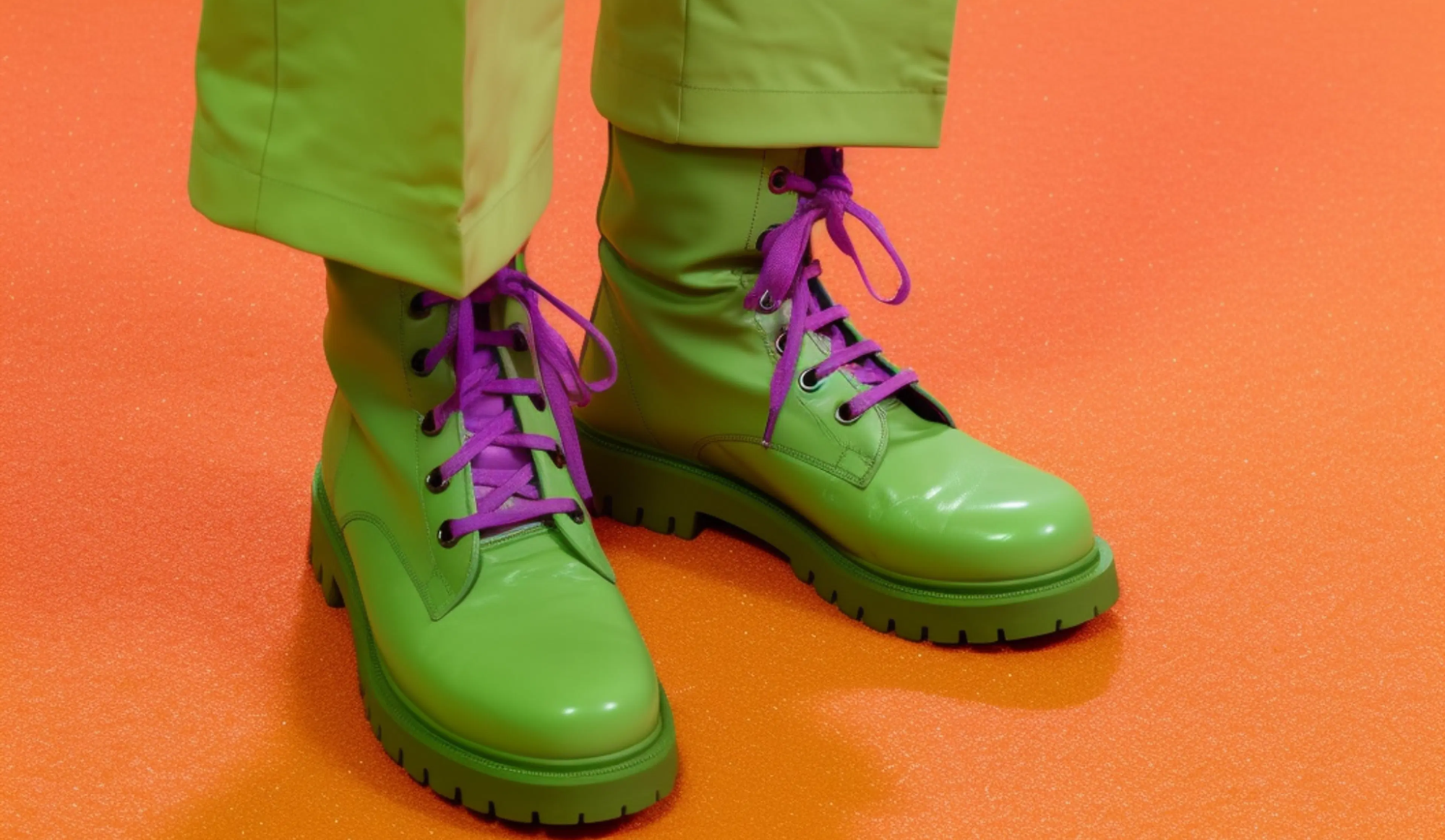
Setting the Stage
Welcome to the thrilling world of Zapatería La Bailarina, where high heels meet high data!
Zapatería La Bailarina, an emerging e-commerce brand in the shoe industry, is navigating the complexities of leveraging data-driven strategies in a competitive market. Establishing an efficient data pipeline, crucial for synthesizing e-commerce transactions, customer interactions, and social media insights, involves intricate complexities. In the bustling e-commerce bazaar, Zapatería La Bailarina is dancing its way through the tango of data-driven strategies. But let’s not sugarcoat it – setting up an efficient data pipeline is like preparing for a grand ball. It’s exciting, essential, and yes, expensive.
This exploration delves into what these challenges mean for Zapatería La Bailarina (let’s call them ZB for short). Let’s peel back the curtain and see what this dance really costs.
Tackling Complexity Head-On
ZB’s data pipeline is built to manage diverse data sources:
Data Warehousing: Opting for a solution like Snowflake, ZB incurs costs based on storage and computing needs, averaging around $3,000 to $5,000 per month.
ETL Tools: Integrating data from their e-commerce platform, customer interactions, and social media analytics with Fivetran adds about $2,000 to $4,000 monthly.
Operational Costs: Navigating Complexity
The operational costs for ZB’s pipeline are influenced by several factors:
Maintenance and Monitoring: A small team of data engineers is necessary for pipeline management, adding roughly $10,000 to $15,000 per month.
Data Integration Tools: ZB uses tools like Informatica for complex data mappings and Stitch for simpler integrations. This mix adds an additional $2,000 to $3,000 to their monthly operational budget.
Understanding the Complexity of Data Tool Pricing
The pricing for ZB’s data tools includes multiple facets:
Monthly Active Rows (MAR): Pricing depends on the number of rows processed each month, fluctuating with seasonal data volume.
Computation and Transformation Costs: These costs arise from processing and transforming data from various sources like online sales, customer feedback, and marketing campaigns.
Loading and Storage Fees: The amount and duration of data storage also impact costs.
Payment Options: ZB weighs between On-Demand (month-to-month) payments for flexibility and Pre-Purchased plans for cost savings but less scalability.
The Impact of Speed and Time-to-Use
Speed in data processing significantly benefits ZB. As Gartner states, “Faster data leads to 2x better marketing outcomes.” This translates to:
Enhanced Customer Experiences: Quick analysis of customer behavior enables personalized interactions.
Agile Decision Making: Rapid data processing allows ZB to swiftly adapt to market trends.
Optimized Marketing Spend: Real-time insights facilitate immediate adjustments in marketing strategies.
Combining these factors, the bottom line for ZB’s monthly data costs ranges from approximately $18,000 to $29,000 per month. This total encompasses warehousing, ETL processing, maintenance, integration tools, and other operational expenses like paying the data team’s salary.
Conclusion: Weighing Costs Against Benefits
Despite significant costs, the investment in a sophisticated data pipeline is justified for ZB by the enhanced marketing effectiveness and operational agility it brings. Understanding the intricacies of data tool pricing, including payment options, and the value of fast data processing is key to making sure ZB stays en vogue in the data-driven world.
Nice. You're now registered for the Lassoo Newsletter.